DeepSeek R1: Revolutionizing AI with Cost-Efficient Reasoning Models
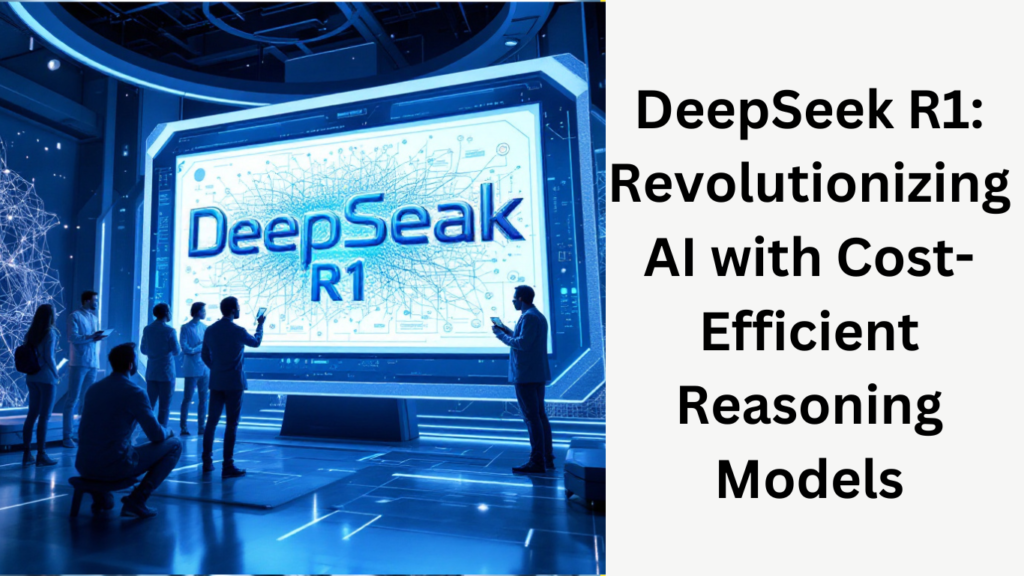
Introduction
In the rapidly evolving landscape of artificial intelligence, DeepSeek, a China-based startup, has made a significant splash with its innovative AI models. DeepSeek R1, their latest offering, has garnered attention by claiming to match or even surpass the performance of industry-leading models at a fraction of the cost. This blog delves into the intricacies of DeepSeek R1, its evolution, and the groundbreaking technologies that make it a game-changer in the AI realm.
The Rise of DeepSeek
DeepSeek’s journey began with the release of its first model, DeepSeek version one, in January 2024. This 67 billion parameter model laid the foundation for future innovations. The subsequent release of DeepSeek version two in June 2024 introduced novel features like multi-headed laden attention and the DeepSeek mixture of experts, enhancing performance and speed.
By December 2024, DeepSeek version three, with 671 billion parameters, incorporated reinforcement learning and optimized GPU usage, setting the stage for the development of reasoning models.
DeepSeek R1: A Breakthrough in AI Reasoning
DeepSeek R1, released in January 2025, is the culmination of DeepSeek’s innovative journey. This reasoning model stands out for its ability to solve complex problems by breaking them down into steps, a process known as chain of thought. Unlike traditional models that provide answers without explanation, DeepSeek R1 offers insights into its reasoning process, making it more transparent and reliable.
Key Features of DeepSeek R1
- Chain of Thought Reasoning: DeepSeek R1 employs a step-by-step analysis process, providing users with a clear understanding of how it arrives at conclusions.
- Reinforcement Learning: The model learns through trial and error, improving its reasoning capabilities over time.
- Mixture of Experts Architecture: This architecture divides the model into specialized sub-networks, activating only the necessary experts for a given task, thus reducing computational costs.
Efficiency and Cost-Effectiveness
One of the most remarkable aspects of DeepSeek R1 is its cost-efficiency. DeepSeek engineers claim that they only need 2000 GPUs to train their models, compared to over 100,000 GPUs used by competitors like Meta for training Llama 4. This significant reduction in hardware requirements translates to a 96% lower cost, making DeepSeek R1 an attractive option for businesses looking to leverage AI without breaking the bank.
How DeepSeek Achieves Cost Efficiency
- Optimized GPU Usage: DeepSeek’s models are designed to balance load across GPUs, ensuring efficient use of resources.
- Reinforcement Learning: By rewarding the model for correctness, DeepSeek R1 discovers the best way to think, reducing the need for extensive labeled data.
- Mixture of Experts Architecture: This architecture not only reduces training costs but also achieves faster performance during inference.
Technological Innovations
DeepSeek R1 incorporates several technological innovations that set it apart from other AI models.
Reinforcement Learning
Reinforcement learning allows the model to improve its reasoning capabilities through trial and error. This approach rewards the model for correct answers, enabling it to learn and adapt over time.
Mixture of Experts Architecture
The mixture of experts architecture divides the model into specialized sub-networks, each focusing on a subset of the input data. This division reduces computational costs and enhances performance during inference.
Distilled Models
DeepSeek also explores the concept of distilled models, where knowledge from a large teacher model is transferred to a smaller student model. This process, known as model translation, allows for the creation of efficient and performant models.
Future Prospects
The future of AI reasoning models looks promising with innovations like DeepSeek R1. As businesses seek cost-effective and efficient AI solutions, models that offer transparency and reliability will be in high demand. DeepSeek’s focus on reinforcement learning and mixture of experts architecture positions it as a leader in this evolving landscape.
Conclusion
DeepSeek R1 represents a significant leap forward in AI reasoning models. Its cost-efficiency, transparency, and innovative technologies make it a compelling option for businesses and researchers alike. As the AI landscape continues to evolve, models like DeepSeek R1 will play a crucial role in shaping the future of artificial intelligence.