Llama 4 Controversy Explained: Is Meta’s New AI Model Overhyped?
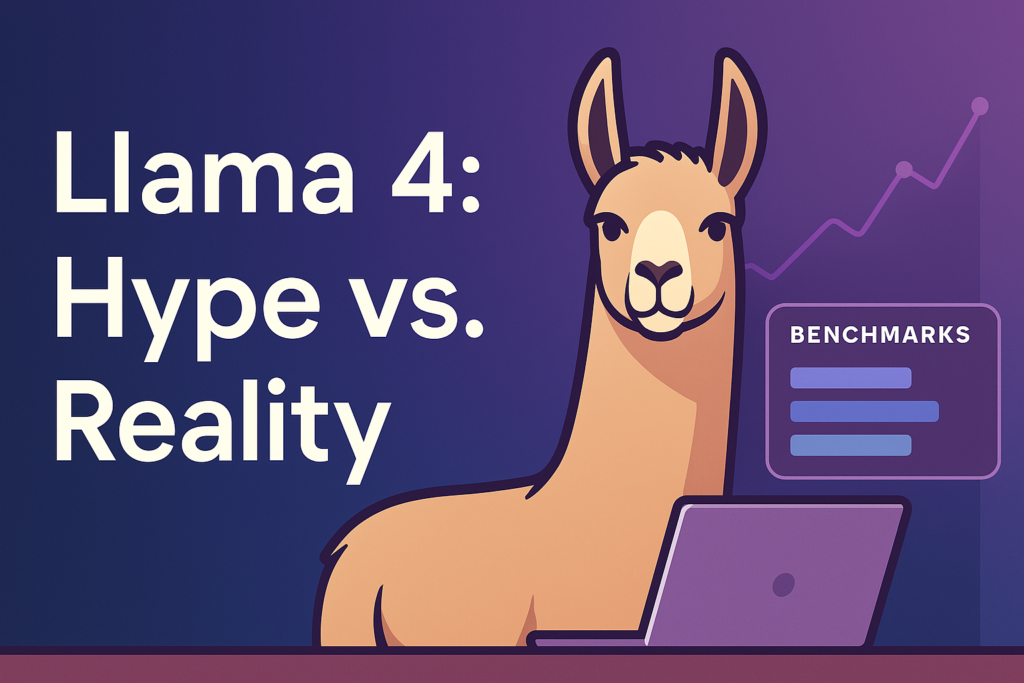
Introduction
The AI world thrives on innovation – and drama. With the release of Llama 4, Meta has found itself in the middle of heated debates around benchmark performance, transparency, and competition with global players like DeepSeek V3. While Meta touts Llama 4 as a powerful step forward, experts and users are questioning the real capabilities of the model.
In this blog, we break down everything you need to know about the Llama 4 release, what’s causing the controversy, and what it means for the future of AI language models.
What Is Llama 4 and Why It Matters
Llama 4 is Meta’s latest large language model (LLM), designed to compete with other top-tier AI models like GPT-4 and Gemini 2.0. With claims of improved accuracy, multilingual capabilities, and better real-world performance, it was expected to be a major breakthrough.
However, the AI community is now asking – does it really live up to the hype?
The Missing Technical Paper
One red flag? Llama 4 was released without a technical paper. While many companies are moving toward closed-source development, the lack of documentation has raised eyebrows.
-
No training data details
-
No architecture disclosures
-
No transparency about evaluation methods
This makes it difficult for developers, researchers, and analysts to understand or trust the model’s performance.
The Benchmark Debate: Real or Rigged?
Critics argue that Meta overfitted Llama 4 to benchmark tests. Ethan Mollick, an AI professor, pointed out discrepancies between the benchmark-winning version and the publicly released model.
For example, when asked the same question, different versions of Llama 4 gave drastically different answers. This suggests:
-
Benchmark scores may have been achieved using a special “Maverick experimental” model
-
The general release may not perform at the same level
-
Benchmark manipulation is possible if only optimized models are tested
This raises serious concerns about how AI model benchmarks are being reported and trusted.
DeepSeek V3: A Disruptive Challenger
Adding to the tension is DeepSeek V3, a Chinese model that reportedly outperformed Llama 4 – with just a $5.5 million budget.
Insider reports suggest Meta’s Gen AI team went into “panic mode”:
-
Engineers were instructed to reverse-engineer DeepSeek
-
Management faced pressure over high salaries compared to DeepSeek’s performance
-
Meta’s internal morale was affected by rapid advancements from lesser-known companies
This shows how quickly the AI race is evolving, with smaller players now threatening tech giants.
Reddit Leaks and Industry Rumors
Several Reddit posts from anonymous insiders hinted at internal chaos within Meta’s Gen AI division. Claims included:
-
Engineers questioning the value of highly paid AI leadership
-
Suggestions to train on benchmark test sets (a major ethical red flag)
-
Resignations over pressure to meet artificial performance targets
Whether fully accurate or not, these leaks matched later public revelations and added credibility to the idea that Llama 4’s release was rushed and pressured.
Meta’s Official Response to Benchmark Concerns
Meta did respond to the growing backlash. Key points included:
-
Acknowledging inconsistent quality across platforms
-
Denying any training on test sets
-
Explaining the need for implementation stabilization
-
Promising transparency through open benchmark battles
They stated, “We believe the Llama 4 models are a significant advancement and are working to unlock their full value with the community.”
Still, doubts remain about the fairness of benchmarking practices and model versioning transparency.
Real-World Testing: Mixed Reactions
AI users have had vastly different experiences with Llama 4:
-
Some found the model great for tasks like social media automation
-
Others reported poor performance compared to competitors in coding and logic
-
Public benchmarks like SEAL LLM flagged potential test set contamination
Depending on use case and platform, performance can vary widely – suggesting that not all Llama 4 versions are created equal.
The Bigger Picture: Transparency in AI
The Llama 4 situation highlights a broader issue in AI:
-
Inconsistent model naming causes confusion
-
Closed-source approaches limit trust and community contribution
-
Lack of benchmark standardization enables manipulation
As AI tools become more integrated into daily life, transparency and ethics become critical.
FAQs
Q1: What makes Llama 4 different from previous versions?
It promises better accuracy, faster response time, and improved performance across languages. But concerns over benchmark manipulation and model versioning have surfaced.
Q2: Is Llama 4 really underperforming?
Depends on the use case. In social tasks, it performs well. In technical tasks, some users report it’s behind GPT-4 and DeepSeek V3.
Q3: Did Meta manipulate benchmarks?
There’s no definitive proof, but side-by-side tests and Reddit leaks suggest the benchmark model may be different from the one released to the public.
Q4: Can I use Llama 4 now?
Yes, through platforms like OpenRouter or poe.com, but expect varying results depending on the version deployed.
Q5: What should users do now?
Stay informed. Use third-party benchmarks, experiment across platforms, and keep an eye on official updates from Meta.